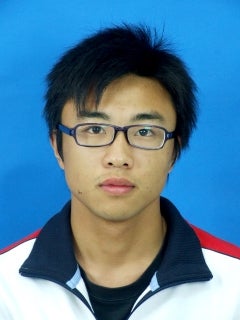
Resume
Education
M.S., | Jiangnan University, | (2017) |
Joint Graduate Student, | Rensselaer Polytechnic Institute, | (2014-2016) |
B.S., | Jiangnan University, | (2011) |
Research Interests
Currently, my research is focused on:
- Building models for soft sensors improve product quality, process safety and to assess the overall process performance of complex chemical production systems. The construction of such models relies on fundamental knowledge about the process in question and recorded data from the process. These models aim to predict process variables that are difficult measure but crucially important, as they directly affect product quality. The literature proposes two distinct approaches for soft sensors: those based upon mechanistic first principle models and those that utilize recorded process variables. Compared to soft sensors based upon mechanistic process models, data driven sensors can reflect current process conditions more accurately with relatively little effort. The disadvantage of data-driven approaches, however, is that they cannot extrapolate, that is accurately predict quality variables from data that are outside the normal operating range, which first principal models (model-based) potentially can. The aim of my research work is to develop approaches that take advantage of benefits of data-driven and model-based techniques.
- Development of nonlinear chemometric latent variable models for process modeling and monitoring. Multivariate statistical process control (MSPC) identifies static or dynamic correlations between variables and generates a reduced set of statistically independent scores that accordingly simplify the monitoring task at hand. At present, MSPC applications are firmly based on principal component analysis (PCA) and partial least squares (PLS) or their extensions as core component technologies. Nonlinear extensions or PCA and PLS have been discussed but are practically difficult to implement, particularly for large scale dynamic systems. Currently, my research focuses on utilizing the fast adaptation of a KPCA/KPLS model to develop a framework for monitoring complex nonlinear systems, selecting the optimal kernel parameter and the number of latent variables and to further develop this into a process monitoring scheme.