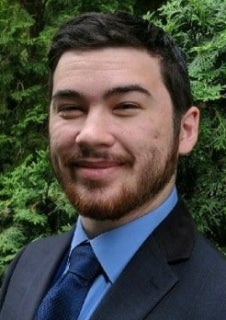
Ph.D., | Rensselaer Polytechnic Institute, | (2024) |
B.S., | Stony Brook University, | (2020) |
Artificial intelligence (AI) and machine learning (ML) models are frequently used to analyze large, complex biomedical datasets. These types of models are commonly used for tasks such as disease diagnosis, biomarker identification, and network analysis. However, the data that these models are derived from and used on are often characterized by significant amounts of noise resulting from patient-to-patient heterogeneity, different measurement protocols, and other commonly encountered sources of noise. This creates a problem for the robustness of these models and one outcome is that relatively few AI/ML models have seen widespread clinical use. As such, evaluation and subsequent improvement of AI/ML model robustness is vital for clinical translation.
This dissertation examines methods which will allow researchers to quantify model robustness, and further demonstrates how to develop more robust AI/ML models. First, this work defines a framework which can be used to evaluate the robustness of an already-trained biomarker-based diagnostic model. This is done by measuring the quality of the biomarkers used to generate the classifier and observing the classifier’s performance when the data is perturbed by several sources of noise. Next, a detailed investigation was performed that looked at the robustness of deep learning medical image classification models in response to being trained by data that was artificially perturbed. One key outcome from this evaluation was that it was demonstrated that perturbing training samples results in excellent classifier performance not only for noisy testing data but also does not sacrifice performance on unperturbed images. This is especially important as a classifier will need to be able to perform well on several distributions of data to truly be generalizable across multiple datasets. Finally, a method for the creation of multi-omic co-expression networks of longitudinal biological data was developed. The robustness of this model was assessed by noise perturbation of the data, and further verified by comparing the model outcomes to known biological information.
By understanding how to measure and improve AI/ML model robustness, robust models can be generated that perform well on diverse sets of data. In conclusion, this dissertation lays the foundation for advancing the clinical applicability of AI/ML models by establishing methodologies to assess and enhance their robustness in the face of inherent data noise.
Union College